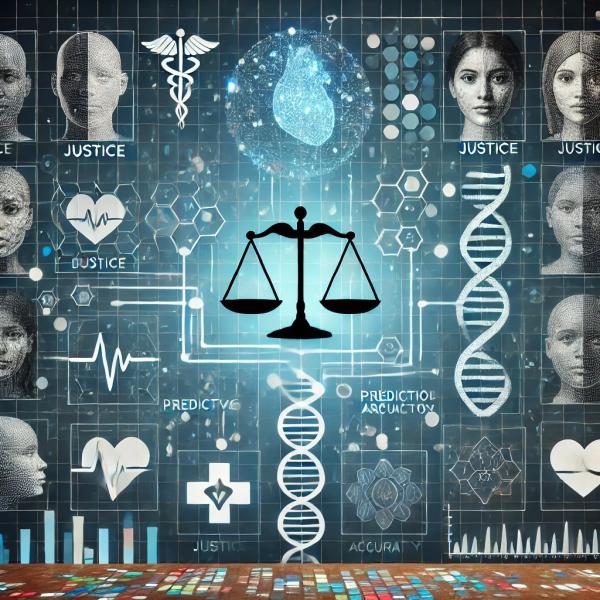
While it is true that racism has ebbed and flowed through medicine, STAT’s Embedded Bias begins by creating a straw man that does not make for the firmest foundation. The article looks at clinical algorithms, tools that help physicians diagnose the presence of a disease identify and quantify risk or project outcomes. Here is the article’s subhead and opening sentence.
“Doctors use problematic race-based algorithms to guide care every day. … a STAT investigation found that race-based algorithms are still widely used across medicine, on millions of patients a year.”
In fact, none of the three clinical algorithms they point to involving measuring kidney function, lung function, or which children may have a urinary tract infection (UTI) are race-based. All of them are statistical associations drawn from the “literature.” A topic STAT rightfully returns to a bit later in discussing that pediatric UTI algorithm.
“When race was put into Boston Children’s UTI calculator, it codified a statistical reality…
Because race information has been collected in medical studies for decades, albeit haphazardly, it often emerges in epidemiological data as a powerful predictor of disease — even when, as in the case of UTI, doctors don’t understand why.” [emphasis added]
Race information is easily measured but rarely defined, making its use problematic. Race has been used as a stand-in proxy for genetics and the social determinants of health. That is quite a load for one term to lift, especially since many of the categorizations of race dealt with superficial phenotypes rather than even the simplest of ethnic and geographical characterizations of Black, Hispanic, Asian, or Indigenous.
“Using race as an input model is kind of lazy” - Maia Hightower, CEO of EqualityAI
Researchers faced with developing clinical algorithms to predict diagnoses, therapeutic outcomes, or risks often use data taken from studies already reported and not directly focused on algorithmic creation. The presence of race is a handy variable for those statistical regressions; given the ambiguous nature of what race means in the context of those studies, it may or may not have clinical applicability.
Consider this hypothetical. Using data from a study of clinical outcomes for men with prostate cancer, I find that Black men have statistically worse outcomes overall, but when categorized by stage and race, outcomes are identical to Whites. Using race in a treatment algorithm might result in the over-treatment of Blacks and be a source of harm. Using race in an access algorithm would identify Blacks with more early-stage, treatable disease with hurdles to access and be a source of benefit. Race serves no clear-cut biological role in my treatment algorithm and should be replaced by stage, but race as a social determinant clearly has a role in access. This fear of “throwing the baby out with the bathwater” is part of what makes Medicine’s response to “race-based” algorithms slow.
“If there are compelling reasons to include race as a variable — which there are — because of the difference in presentation, difference in prognosis, sometimes differences in therapy, then it’s going to be included.”
-Debra Patt, MD Member Board of the American Society of Clinical Oncology.
The Agency for Healthcare Research and Quality (AHRQ) released its report on algorithm-related racial and ethnic disparities in healthcare – a meta-analysis of 17 studies on the impact of 18 algorithms [1] on racial and ethnic disparities in health and healthcare. They found that algorithms:
- Could reduce disparities (5 studies), exacerbate or perpetuate them (11 studies), or have no effect (1 study)
- Three of the four algorithms that included race-reduced disparities
- In cases like the revised Kidney Allocation System, race and ethnicity were intentionally included to address existing disparities.
Paved with Good Intentions Do No Harm
Do No Harm is one of the ethical pillars of medicine, but as STAT points out, it is often a double-edged sword. Ironically, race was introduced partly to reduce the harm of a one-size-fits-all view of health. As STAT writes,
“Until the National Institutes of Health began mandating the collection of race data and set standards for diversity in clinical trials in the 1990s, disease risk predictions defaulted to reflect the white populations that dominated research. Adding race to the equation, they thought, would help move from whitewashed medicine toward more personalized care and help curb disparities.”
Leadership is scarce.
STAT does point to a critical problem in improving our algorithms – the downstream of Do No Harm are the halting steps, if any, taken by medicine’s guilds, e.g., the American College of Cardiology or the American Academy of Pediatrics. And if the government’s carrots, i.e., funding for studies that would provide more and better data, have evidenced little interest, the Department of Health and Human Services is all set to apply a stick. In May 2025, a new rule:
“prohibits discrimination through the use of patient care decision support tools, including commonly used calculators, and requires care providers to take steps to identify and fix discriminatory tools.”
The wording seems to favor voluntary self-regulation rather than outright penalties. Why some feel self-policing is sufficient for health systems but insufficient for commercial, corporate entities is unclear. Especially given this:
“Accountability is … hugely lacking, both the carrot and the sticks,”
- Michelle Morse, chief medical officer, NYC Health Department, leader of the NYC Coalition to End Racism in Clinical Algorithms (CERCA)
“We have intentionally stayed away from this topic as a research one and let the societies and the clinical scientists figure it out,”
- Eliseo Pérez-Stable, director of the National Institute on Minority Health and Health Disparities
STAT ends on this note, framing the clinical desire for race-free tools that “won’t lose their predictive power or harm patients in unforeseen ways.” However, race-free is not a requirement to be predictive and not harmful. This final framing is, for me, the most contentious.
“In the absence of conclusive answers, clinicians and medical societies are left to make a value judgment, choosing between maximum predictive accuracy or racial justice.”
Social justices may be part of my duty as a citizen, but in the moment of the physician-patient relationship, my duty to my patient is predictive accuracy; racial justice is not part of the calculus.
Ultimately, race-based algorithms aren’t as simple as “good” or “bad” – they exist in a medical gray area where their utility depends on context. We must be cautious not to vilify them entirely without considering the nuances and complexities of medical decision-making. While racial bias in healthcare, as in most human behavior, is real, algorithms alone aren’t the culprit; they are tools that need careful calibration and context. Instead of discarding them altogether, we should focus on refining their use, ensuring they are informed by science and equity. After all, good intentions are meaningless without careful execution.
[1] The algorithms were primarily used to guide resource allocation during crises, inform emergency department care, and determine eligibility for lung and prostate cancer screenings. None of the studies were randomized controlled trials.