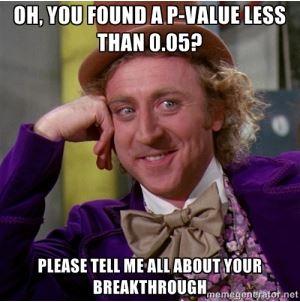
In science and health, we are often looking for results that are considered to be “statistically significant.” The golden rule is if the p-value is less than 0.05, then the result is statistically significant, or “publishable.” However, the interpretation and use of p-values is often misconstrued.
What is a p-value?
A p-value is the probability of observing a test statistic as extreme as the data shows, given that the null hypothesis is true. It does NOT tell you what the probability of the hypothesis is given the data. Confusing these two is equivalent to mixing up “given that someone is Catholic, what is the probability that they are the Pope?” and “given that someone is the Pope, what is the probability that they are Catholic?”
How are p-values useful?
P-values help you make a decision regarding the null hypothesis. A null hypothesis states that there is no difference between specified populations, and that any observed difference can be attributed to sampling or experimental error. A small p-value indicates that there is strong evidence against the null hypothesis, and indicates that you should reject this claim in favor of the alternative. The alternative hypothesis rivals the null, stating that the observations are the result of a real effect.
Problems with p-values
While p-values can be very useful in statistics, they can also be manipulated. p-hacking (data dredging) and p-HARKing (hypothesis after results are known) are processes by which researchers collect or select data that make nonsignificant results become significant.
Influencing data to make it significant, or to draw a hypothesis after you already know the results, is just bad science. It is the responsibility of the scientific community to know what a truly significant p-value looks like, and more importantly, what it means.