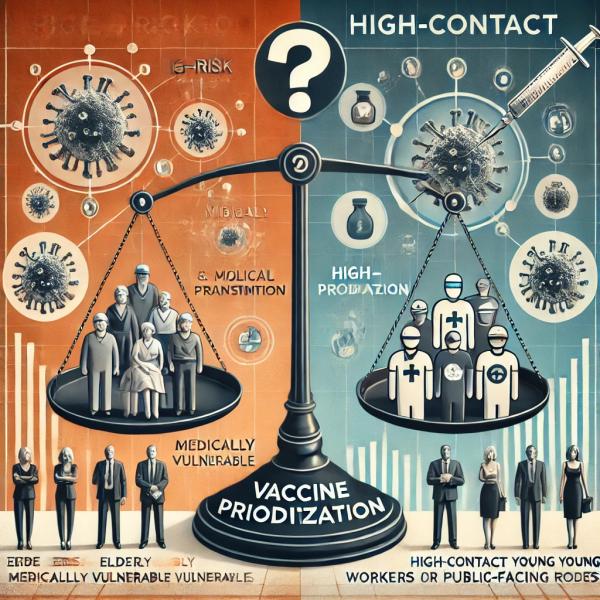
There is little doubt that we might have handled the COVID pandemic better. However, the question of whether the approach by those writing the Barrington Declaration would have fared better remains conjecture. In the face of a novel infection, public health faced the task of identifying those at risk and the carriers, limited by the efficacy of their tools in reducing the prevalence and severity of illness. A modeling study in PLOS One tries to identify optimum strategies because one-size-fits-all is rarely optimal.
Developing an optimum strategy
The researchers, modeling the COVID-19 pandemic, assumed a vaccine's availability, initially in limited doses, as was the case due to Operation Warp Speed. If you remember, the limited vaccine prompted prioritizing some groups over others based on differences in how much they interact and their risk of severe illness. With limited doses, authorities could choose to vaccinate:
1. High-risk group: Elderly and immunocompromised individuals, reducing severe illness and death.
2. High contact group: Those with many daily interactions (e.g., shopkeepers, drivers) curbing transmission.
3. A mix of both: Combining protection for the vulnerable and reducing spread.
As the researchers point out, our public health approach prioritized those at high risk over high contact, except first responders, who were “a negligible segment of the high contact group.” However, what if prevention, by vaccinating the high-contact “spreaders,” was more effective – a case that might be especially true if the high-contact spreaders were just a tiny fraction of the population, making quick coverage feasible?
There was also discussion about when the second dose of vaccine should be administered. With a limited supply should we give everyone some protection or some complete protection? Or would a hybrid strategy be more optimal?
Finally, we need to acknowledge that
- COVID-19’s pathogenicity was novel. Unlike influenza or other viral illnesses, COVID-19 disproportionately harms the elderly and those with conditions like diabetes, obesity, and hypertension—people over 65, for example, face dramatically higher risks, over 5-fold, and made up 81% of US COVID deaths in the first year.
- Much of the narrative and public understanding of vaccines is based on one-and-done illnesses, like measles, or assumes uniform risk – assumptions that COVID-19 did not meet.
Modeling the Public Health Response
Given the “multi-dimensional heterogeneity” of COVID-19, researchers created population groupings based on their risk and contact rates, which varied according to age and occupation. In making these groupings, the researchers traded the analytics gained against the need for greater and greater computation time to ascertain that information.
The modeled goal of vaccination was improving “public health outcomes like reducing deaths, hospitalizations, and symptoms” while accounting for vaccination capacity, defined as vaccination supplies and the labor force necessary to give those vaccinations.
After some initial pilot studies, the researchers found that the optimal vaccination strategies could be reduced to a handful.
The populations
Different groups of the populace constitute the most potent vector of spread, while others have a high risk of developing a severe disease once infected. The researchers defined three groups:
Baseline - those who are neither high contact nor high risk, having small sets of “regular” contacts, e.g., kids, family members, colleagues, or friends, with whom they connect frequently. However, outside this set, they infrequently connect with others.
High risk - those who are old or have underlying health conditions, exacerbating the risk of developing symptoms, hospitalization, and death once infected, e.g., retirees
High contact - those who have overall high rates of contact with both of the other groups. Given their large and dynamic contact sets, individuals in this group are the most likely to be exposed to an infectious disease early and pass the same to a large number, e.g., nursing home workers and first responders. This group constitutes the most potent vector of spread.
Vaccination
The model tracks COVID-19 progression using a 10-state compartmental system [1]. Vaccination is prioritized for susceptible individuals, following CDC guidelines, to maximize effectiveness, especially in resource-limited settings.
Vaccination capacity is limited by available healthcare workers, infrastructure, and cold storage constraints, restricting the number of doses that can be administered daily. The optimal vaccination strategy minimizes the overall death count and satisfies the vaccination capacity constraint.
There were two candidate strategies for one-dose vaccines. The first strategy involves vaccinating individuals with high risk first, then moving on to those at high contact risks, and finally addressing the baseline group. This seems intuitive, targeting the most vulnerable. However, the researchers found that in many realistic settings, such as nursing homes or in low-to-middle-income countries (LMIC), prioritizing the high-contact group, then the high-risk, and finally the baseline could lead to lower death counts. Remarkably, high-risk or high contact first consistently optimizes key public health outcomes dependent upon other factors, stressing the need for more localized, precise strategies.
For two-dose vaccines, the optimal strategies differ in the order in which groups are vaccinated and whether the second dose is delayed until everyone has received the first dose or given in quick succession within each group while others wait. The analysis found no need to split vaccine doses between groups. An optimal strategy uses full vaccine capacity on one group at a time. Once the number of unvaccinated individuals in the prioritized group falls below vaccination capacity, would the optimal strategy consider vaccinating two groups simultaneously.
Model validation
The model was validated using real-world data to predict the spread and mortality of COVID-19 across all US states and 139 countries. The graphic shows the results for three areas: California, Florida, and Bangladesh. Florida has a significantly greater population of elderly and a more laissez-faire approach to social masking and distancing. California was younger and stricter, while Bangladesh was chosen as an exemplar of an LMIC, with a significantly younger population and widely different governmental responses than the US.
“the average difference between the predicted and actually recorded values is less than 1% for both infections and death.”
Findings
- “In 93.4% of our cases, the best strategy was simple: either fully vaccinate the high-contact group first, then the high-risk group (high-contact prioritization), or do the reverse (high-risk prioritization), with the baseline group always vaccinated last.”
- The researchers asked if these simple policies would alter mortality if they were more or less dynamic, comparing an unchanging vaccination strategy with one that varied over monthly time frames. (You might remember the attempt at dynamic, focal neighborhood lockdowns in New York City). Static policies were not as effective as even the most minimally dynamic ones, sometimes resulting in three times as many deaths. While a monthly adjustment policy performs better than an utterly static approach, both still
lag behind the fully dynamic strategy, underscoring the need for a flexible vaccine allocation plan in rapidly changing conditions. While the graphic depicts “how mortality substantially decreases as we increase the number of decision intervals,” logistical considerations may make this impractical.
While public health authorities mainly used a high-risk prioritization strategy, including early vaccination for first responders, high-contact prioritization was, in fact, better when considering the three major COVID-19 variants: Alpha, Delta, and Omicron [2]. For example, Omicron’s higher transmissibility made high-contact vaccine prioritization more beneficial. But that benefit is lost when the initial level of infection is high because while you are busy vaccinating the high contact, the high risk is getting infected and dying. Infectivity, R0, makes a difference, and it took some time to determine Alpha’s R0.
“Overall, the numerical computations reveal that, while the common high-risk priority policy is optimal in the majority, there is a considerable range of realistic parameters for which high contact prioritization more effectively lowers mortality.”
- High-risk groups drive mortality rates; high-contact groups drive rates of infection. To reduce deaths, high-risk prioritization is best; to reduce infections a high-contact strategy.
- In most instances, about 69%, the optimal two-dose vaccination strategy involves giving both doses to a group without any delay between doses. If the first dose provides sufficient protection, a delayed second dose strategy reduces mortality and infections, easing the overall burden on health systems and the economy. However, in focusing on high-risk individuals, immediate complete protection is necessary.
- Vaccine efficacy is critical; some protect, reducing disease severity; others prevent, lowering infectious risk. The best do both. COVID vaccines were protective but were less effective at prevention. When protection efficacy is high, protection-focused vaccines perform better; when protection efficacy is low, prevention-focused vaccines lower deaths more.
- Total deaths increase as contact rates rise. When contact rates are very low, the disease spreads slowly, so targeting high-contact individuals adds little benefit; when rates are high, the virus spreads too quickly for this approach to be practical. High contact prioritization works best at moderate contact levels.
If the COVID-19 pandemic taught us anything, it’s that public health strategies are only as good as the assumptions behind them. While prioritizing the high-risk group made intuitive sense, this study highlights a significant flaw in that approach—when transmission is fueled by a small, highly interactive population segment, targeting them first can sometimes be the better move. High-contact prioritization isn’t just a theoretical exercise; in many scenarios, it significantly reduces mortality compared to the traditional high-risk-first strategy.
The lesson here is clear: rigid, one-size-fits-all vaccine rollouts fail to account for the dynamic nature of disease. The best strategies adapt to real-world conditions—whether it’s the emergence of a more transmissible variant, the size of the high-contact population, or the effectiveness of a single vaccine dose. Data, not dogma, should guide public health policy. Because in a pandemic, the difference between a good strategy and the right strategy isn’t just a statistic—it’s lives saved.
[1] “susceptible (S), exposed (E), pre-symptomatic (P), asymptomatic (A), early-stage infected (I), late-stage infection (L), hospitalized (H), recovered (R), vaccinated (V), and dead (D).”
[2] Alpha, the original; Delta, nearly twice as contagious and double the hospitalization risk of Alpha; and Omicron, more infectious than Alpha but with lower mortality rates.
Source: Protect or prevent? A practicable framework for the dilemmas of COVID-19 vaccine prioritization PLOS One DOI: 10.1371/journal.pone.0316294